About
Official page of the Madrid meetup of the Learning on Graphs Conference, an annual research conference that covers areas broadly related to machine learning on graphs and geometry.
The event serves as a local branch of the main conference, providing an environment for researchers in this field to convene and foster discussion and social connections.
Explore the event's recorded sessions here.
📅 27th – 29th November 2023 📍 Madrid
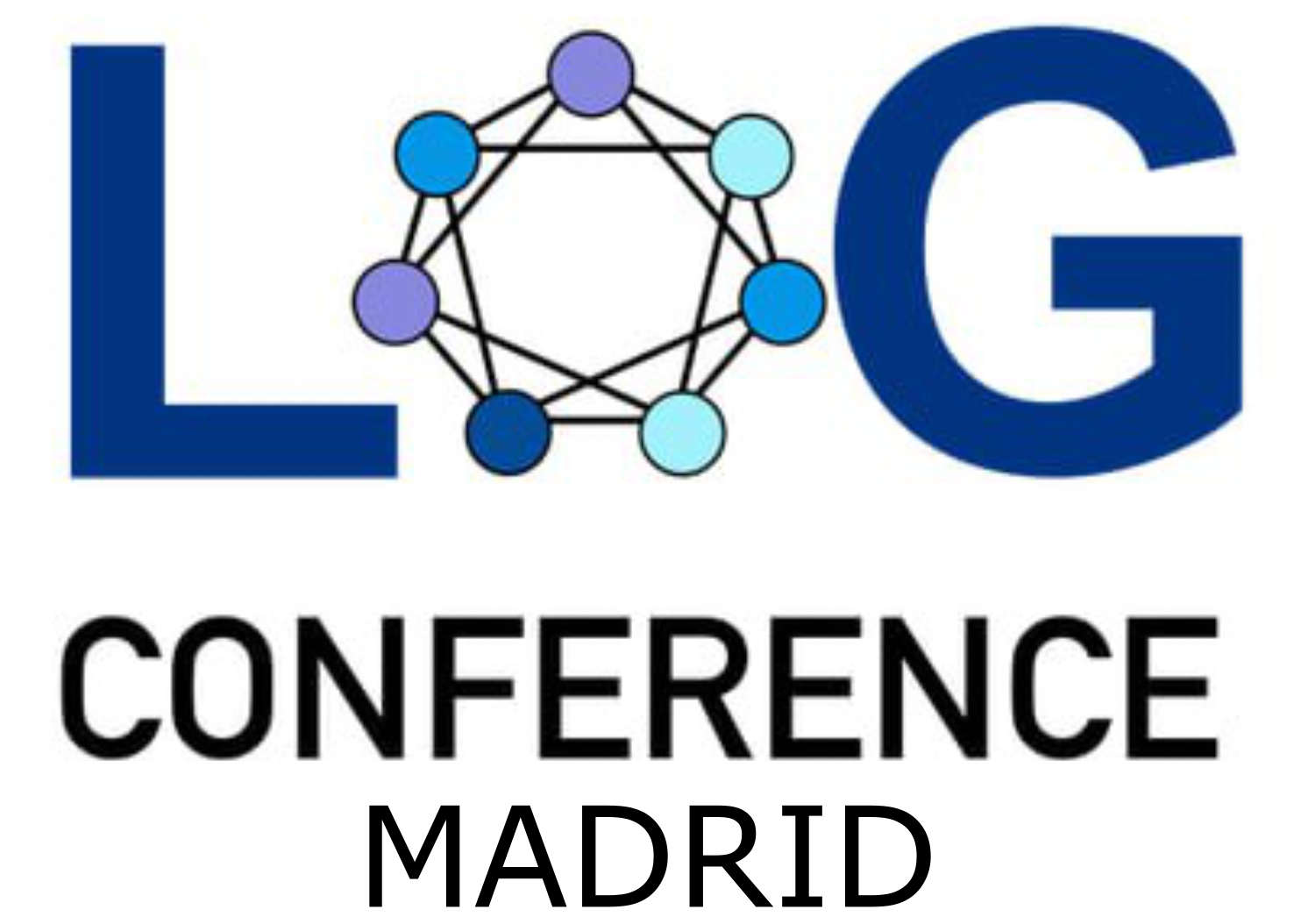
Important dates:
- Submission deadline: November 8th 2023
- Final decision: November 10th 2023
- Deadline for registration: November 19th 2023
- Late registration until: November 26th 2023
- Late full registration fee: 180€.
- Reduced late full registration fee (students and part-time attendees with a talk): 90€
Registration
If you are interested in participating or attending the Meet Up, you can register until November 19th, 2023, by filling out this form. Note that at least one author of each accepted submission has to register and attend the workshop in person. We have set a low early registration fee of 30€ per person to cover expenses. Registration fee 180€, with a reduced of 90€ for students and part-time attendees with a talk.
We also encourage all LoG@Madrid participants to register for the main LoG conference. Check the information available on Learning on Graphs Conference.
Call for talks
We are planning a session of open talks on general topics and open problems related to learning on graphs and geometry. Authors whose abstracts are accepted to the workshop will present their work to the full audience. The best submissions will be chosen for oral presentation.
We kindly request authors to provide an abstract of a maximum of 4000 characters (approximately 600 words). If you have recently published papers relevant to the conference theme, please submit your abstract along with their respective DOIs through the submission form. Further, if your work belongs to one of the subject areas below, please specify on the form.
Talks abstracts must be submitted through the submission page.
In the case that the number of applications should exceed the number of talks that can be included in the session, the organizing committee will reserve the right to select a limited number of talks. More information on timing will be released as the schedule of the main conference is made known.
Subject Area
The following is a summary of LoG’s focus, which is not exhaustive. If you doubt that your paper fits the venue, feel free to contact logmeetupmadrid@gmail.com!
- Expressive Graph Neural Networks
- GNN architectures (transformers, new positional encodings, ...)
- Statistical theory on graphs
- Causal inference (structural causal models, ...)
- Geometry processing
- Robustness and adversarial attacks on graphs
- Combinatorial Optimization and Graph Algorithms
- Graph Kernels
- Graph Signal Processing/Spectral Methods
- Graph Generative Models
- Scalable Graph Learning Models and Methods
- Graphs for Recommender Systems
- Knowledge Graphs
- Graph ML Platforms and Systems
- Self-supervised learning on graphs
- Trustworthy graph ML (fairness, privacy, …)
- Graph/Geometric ML for Health, Security, Computer Vision, ...
- Graph/Geometric ML Infrastructures (datasets, benchmarks, libraries, …)
Schedule
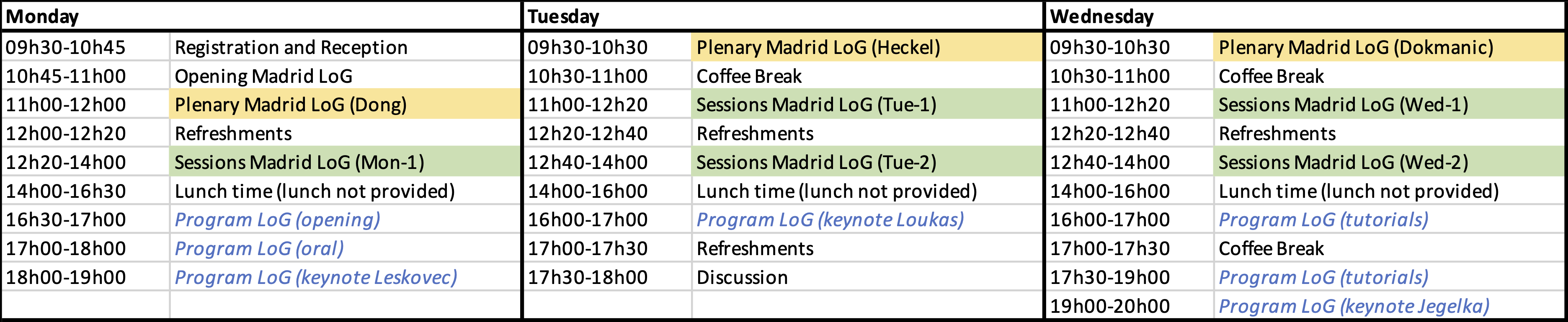
Download a PDF version of the schedule here.
The details for each presentation slot, encompassing presenter names and their respective talk titles, will be made available shortly.
Time | Slot |
---|---|
Monday 9h30-10h45 | Registration and Reception |
Monday 10h45-11h00 | Opening Madrid LoG |
Monday 11h00-12h00 | ![]() |
Monday 12h00-12h20 | Refreshments |
Monday 12h20-14h00 | Sessions Madrid LoG (Mon-1) |
Monday 14h00-16h30 | Lunch time (lunch not provided) |
Monday 16h30-17h00 | Program LoG (opening) |
Monday 17h00-18h00 | Program LoG (oral) |
Monday 18h00-19h00 | Program LoG (keynote Leskovec) |
Tuesday - 09h30-10h30 | ![]() |
Tuesday - 10h30-11h00 | Coffee Break |
Tuesday - 11h00-12h20 | Sessions Madrid LoG (Tue-1) |
Tuesday - 12h20-12h40 | Refreshments |
Tuesday - 12h40-14h00 | Sessions Madrid LoG (Tue-2) |
Tuesday - 14h00-16h00 | Lunch time (lunch not provided) |
Tuesday - 16h00-17h00 | Program LoG (keynote Loukas) |
Tuesday - 17h00-17h30 | Refreshments |
Tuesday - 17h30-18h00 | Discussion |
Wednesday - 09h30-10h30 | ![]() |
Wednesday - 10h30-11h00 | Coffee Break |
Wednesday - 11h00-12h20 | Sessions Madrid LoG (Wed-1) |
Wednesday - 12h20-12h40 | Refreshments |
Wednesday - 12h40-14h00 | Sessions Madrid LoG (Wed-2) |
Wednesday - 14h00-16h00 | Lunch time (lunch not provided) |
Wednesday - 16h00-17h00 | Program LoG (tutorials) |
Wednesday - 17h00-17h30 | Coffee Break |
Wednesday - 17h30-19h00 | Program LoG (tutorials) |
Wednesday - 19h00-20h00 | Program LoG (keynote Jegelka) |
Keynote Speakers
Monday 11h00-12h00 Xiaowen Dong
On the stability of spectral graph filters and beyond: A topological perspective.
Abstract: Graph-based signal processing and machine learning are recent techniques that have been developed to handle graph-structured data and have seen applications in such diverse fields as drug discovery, fake news detection, and traffic prediction. Despite the encouraging empirical results, a theoretical understanding of the robustness of these models against perturbation to the input graph domain has been lacking. In this talk, I will present our results on the stability bounds of spectral graph filters as well as other recent work on the robustness of graph machine learning models. A commonality of these studies is that they all share a topological perspective, that is, linking robustness to topological properties of the graph domain and perturbation. This contributes to a better understanding of robustness hence the deployment of graph-based models in real-world scenarios.
Biography: Xiaowen Dong is an associate professor in the Department of Engineering Science at the University of Oxford, where he is a member of both the Machine Learning Research Group and the Oxford-Man Institute. Prior to joining Oxford, he was a postdoctoral associate in the MIT Media Lab, where he remains as a research affiliate, and received his PhD degree from the Swiss Federal Institute of Technology (EPFL), Lausanne, Switzerland. His main research interests concern signal processing and machine learning techniques for analysing network data, and their applications in social and economic sciences.
Tuesday - 09h30-10h30 Reinhard Heckel
Unpacking Data Needs for Deep Learning Based Imaging
Abstract: Deep neural networks trained on example images enable a new generation of consumer, medical, and scientific imaging systems. I will start this talk with highlighting the role of training data. Surprisingly little data is needed for obtaining state-of-the-art performance. Moreover, it is possible to learn effectively without clean ground-truth data with self-supervised learning methods. However, the excellent performance of deep networks for imaging can be deceiving, as it is usually measured by training and testing on similar looking data, say on data from one hospital. By evaluating neural networks in a variety of practical scenarios, for example by training and evaluating networks on data from different hospitals, we identified a key challenge in deep learning based imaging: robustness to distribution shifts. Finally, I will discuss model and data driven approaches for building robust deep learning based imaging methods.
Biography: Reinhard Heckel is a Rudolf Moessbauer assistant professor in the Department of Computer Engineering at the Technical University of Munich, and an adjunct assistant professor at Rice University, where he was an assistant professor in the ECE department from 2017-2019. Before that, he was a postdoctoral researcher in the Department of Electrical Engineering and Computer Sciences at the University of California, Berkeley, and a researcher at the Cognitive Computing & Computational Sciences Department at IBM Research Zurich. He completed his PhD in electrical engineering in 2014 at ETH Zurich and was a visiting PhD student at the Statistics Department at Stanford University. Reinhard is working in the intersection of machine learning and signal/information processing with a current focus on deep networks for solving inverse problems, developing foundations and methods for machine learning, and DNA data storage.
Wednesday - 09h30-10h30 Ivan Dokmanić
Statistical Mechanics of Graph Convolution Networks
Abstract: Graph neural networks (GNNs) excel in modeling relational data such as biological, social, and transportation networks, but the underpinnings of their success are elusive. Traditional complexity measures from statistical learning theory fail to account for observed phenomena like the double descent or the impact of relational semantics on generalization error. Motivated by experimental observations of ``transductive'' double descent in key networks and datasets, we use analytical tools from statistical physics and random matrix theory to precisely characterize generalization in simple graph convolution networks on the contextual stochastic block model. Our results illuminate the nuances of learning on homophilic versus heterophilic data and predict double descent whose existence in GNNs has been questioned by recent work. We show how risk is shaped by the interplay between the graph noise, feature noise, and the number of training labels. Our findings apply beyond stylized models, capturing qualitative trends in real-world GNNs and datasets. As a case in point, we use our analytic insights to improve performance of state-of-the-art graph convolution networks on heterophilic datasets.
Biography: Associate Professor at the Department of Mathematics and Computer Science of the University of Basel.
Selected Talks
Monday-1 12:20 - 14:00
Learning Types of Hierarchical Relationships on Complex Class Labelling Systems - Speaker: Connor Clarkson
Structure-Preserving Graph Neural Networks - Speaker: Alberto Badias
ResolvNet: A Graph Convolutional Network with multi-scale Consistency - Speaker: Christian Koke
Robust Graph Neural Network based on Graph Denoising - Speaker: Samuel Rey
Learning Graphs and Simplicial Complexes from Data - Speaker: Andrei Buciulea Vlas
Tuesday-1 11:00 - 12:40
Towards a more inductive world for drug repurposing approaches - Speaker: JesĂşs de la Fuente
Leveraging Temporal Graph Neural Networks for Drug Repurposing Using Prescription Data in Norway - Speaker: Asieh Abolpour Mofrad
A molecular foundation model for drug discovery based on molecular graphs - Speaker: Oscar Mendez-Lucio
GeNNius: An ultrafast drug-target interaction inference method based on graph neural networks - Speaker: UxĂa Veleiro
Tuesday-2 12:40 - 14:00
A General Graph Convolution Theorem: Application to Dual Graph Inference - Speaker: Geert Leus
Tensor completion using graph-based diffusion: a remote sensing image completion study - Speaker: Iain Rolland
Recovering Missing Node Features with Local Structure-based Embeddings - Speaker: VĂctor M Tenorio
Completing Missing Air Quality Data with Graph-Based Techniques - Speaker: Michelle Wan
Wednesday-1 11:00 - 12:40
Using Graph Neural Networks to Predict Airport Congestion and Air Traffic Delays - Speaker: Guillermo MegĂas
Spatio-Temporal Graphs and GNN for Antimicrobial Multidrug Resistance Prediction in Intensive Care Unit - Speaker: Oscar Escudero
gMCSpy: Efficient and accurate computation of Genetic Minimal Cut Sets in Python - Speaker: Carlos J Rodriguez
Bayesian Graph Neural Networks, how to optimize a cellular network and provide confidence to our customers - Speaker: Oscar Llorente Gonzalez
Wednesday-2 12:40 - 14:00
Asymmetry-Labeled DAGs: Representation, Learning and Causal Reasoning - Speaker: Manuele Leonelli
HoloNets: Spectral Convolutions do extend to Directed Graphs - Speaker: Christian Koke
Graph-Based Dimensionality Reduction and Clustering for Earth and Life Sciences - Speaker: Nathan Mankovich
Organizers
Local Team
- Andrei Buciulea
- Óscar Escudero
- Sergio Rozada
- VĂctor Tenorio
- Bianca Radu
- Fernando Real Rojas
Sponsors
The Venue
The Madrid LoG Meet Up will take place in the “URJC Madrid-Arguelles Campus”, in one of the buildings that the King Juan Carlos University has in Madrid Downtown.
The location and street view of the building are shown below:
The building has two main entrances. Either one will work but the one in Calle Ferraz 21, Madrid, will give direct access to the Meet Up location.
For those staying around the Plaza de España area, walking to the conference venue will take between 5 and 10 minutes.
Those staying in other places of the city can use the subway system (locally referred to as “El Metro”). The closest metro stations are ArgĂĽelles (Lines 3/4/6) and Ventura RodrĂguez (Line 3). The walking distance from any of these two stations to the conference is less than 3 minutes.